Vibe-coding workflows 🪄
A top-down process for doing AI coding right, plus a full cast of supporting techniques.
Hey everyone! Our most popular article over the last few months is—maybe unsurprisingly—about how to use AI in engineering teams.
It got an awesome reception, but it was mostly a qualitative piece, giving you direction and trying to predict what comes next.
So, after that, many of you reached out via email asking for more. In particular, you asked for real world use cases, complete with prompts and everything!
Today we are doing just that, by bringing in Justin Reock, deputy CTO at DX, who analyzed data from DX customers about GenAI tool usage, use cases, and impact. Justin and his team also collected insights from interviews with S-level leaders who have successfully rolled out AI code assistants to thousands of developers.
The result is a list of the ten most impactful AI use cases in engineering, based on a survey of developers who have self-reported at least an hour of time savings a week using AI assistants 👇
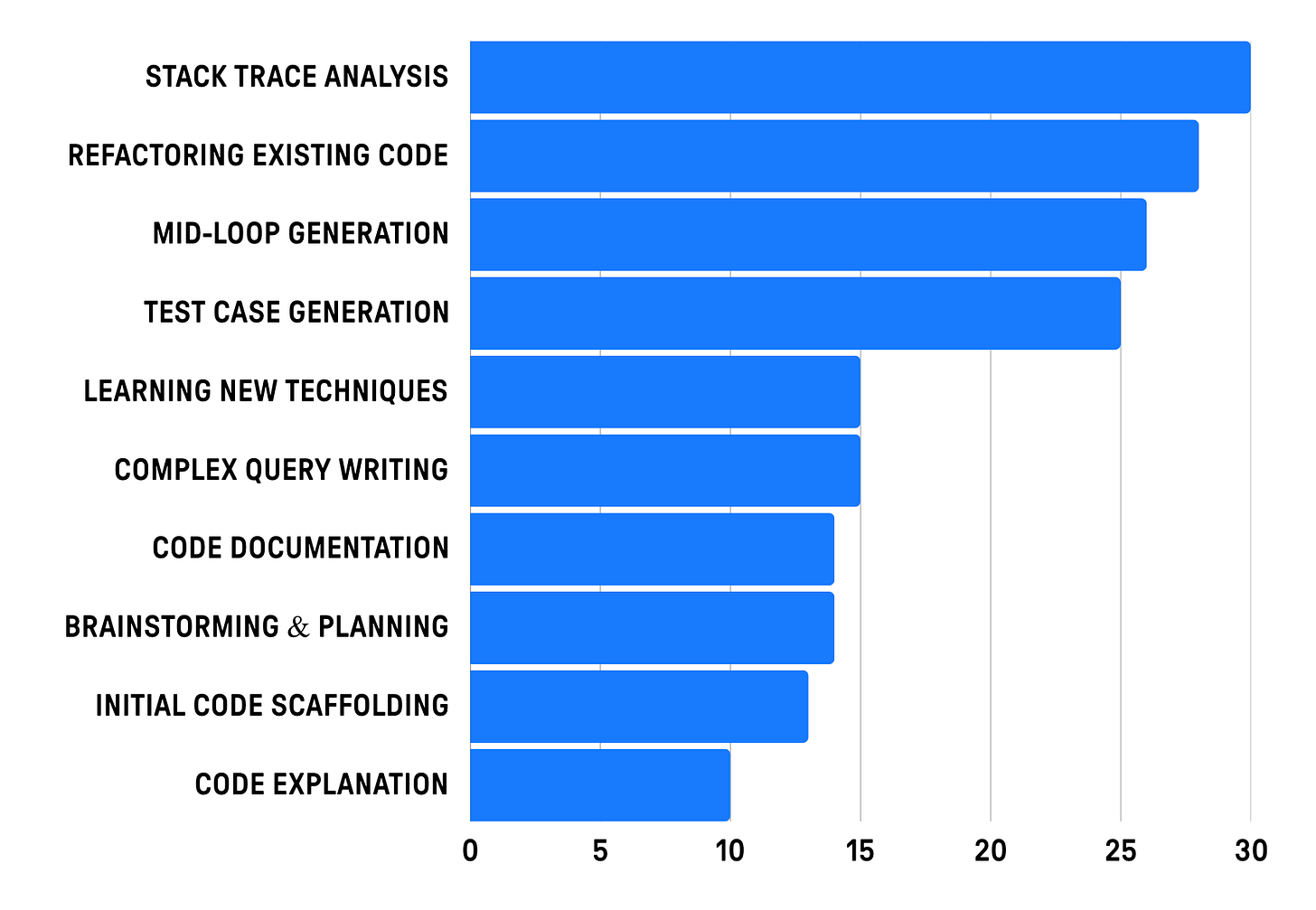
Today we are covering all these techniques, including examples and prompts. We are also going beyond the mere list: we are using this as a starting point to synthesize a basic workflow for vibe-coding successfully.
Here is the agenda:
🗺️ Vibe-coding workflow — how to do it right by working top-down, optimizing cognitive load, and intercepting errors at the right level.
🛠️ Supporting techniques — a tool belt of ideas to leverage AI across the whole coding spectrum.
Let’s dive in!
🗺️ Vibe-coding workflow
Using generative AI can be incredibly effective to plan out and implement entire projects — but it can also make you run in circles and eventually lose time with respect to doing the work by yourself.
The difference between a successful and an unsuccessful output often lies in good prompting, and, even more, in your prompting workflow.
For new developments, like new features or entire small projects, we believe that the most effective workflow is a top-down interaction that goes through several steps.
You can begin with a discussion about your work requirements, and, step by step, get to high-level system design, the full code spec, classes and functions scaffolding, up to the fully coded version.
Working top-down (as we will see in the example) is incredibly helpful in keeping us — humans — engaged in the work. It optimizes our cognitive load and allows us to intercept errors at the right level. With that respect, there is little difference between designing software with or without AI: you just don’t want to rectify system design elements, or requirements misunderstandings, by the time you already have the full code — regardless of whether it was written by a human or an AI.
Still, many vibe-coding examples online dive code-first into AI implementation, which is easily overwhelming for humans and more prone to errors.
So let’s see how this works in a real-world example.
1) 🧠 Brainstorming & Planning
For an initial requirements brainstorming, you can use a simple prompt like this:
Example prompt
I am a product manager and you are a senior software architect. Let's have a brainstorming session where you ask me one question at a time about these requirements, and come up with a specification that I can hand to a software developer.
I want to design an application that will create an ElasticSearch index for a large table stored in Cassandra. Help me design a bulletproof, zero-loss system to do this.
Example output
Great! Let’s break this down systematically. I’ll start with foundational questions, then dive deeper into specific requirements and constraints.
Question 1: What is the primary use case for this ElasticSearch index?
Are we optimizing for full-text search, fast lookups, analytics, or something else?
Will the data in Cassandra be static, append-only, or frequently updated?
How large is the dataset (number of rows, data volume, expected growth)?
This will guide our approach to indexing, data modeling, and ensuring a zero-loss system.
These initial steps benefit from a number of back-and-forth questions where you get to detail what you have in mind. About this, remember that voice prompting is faster than typing, and less cognitively intense! You can use that especially at this stage.
Once you’ve engaged in enough questions, the AI will let you know that you are moving into final questions, and may produce a summary similar to this:
Your system is now:
Auto-Scaling (Kafka, ElasticSearch, Cassandra)
Fault-Tolerant with Multi-Region Replication
Zero-Loss with At-Least-Once Processing & Dead Letter Queue
Real-Time Indexing with Bulk Writes
Enterprise-Grade Security (RBAC, Encryption, SIEM Logging)
Disaster Recovery Across Regions with Failover Handling
Given a similar prompt to the one above, the assistant will then ask if you are ready to create a code spec based on these answers, and whether there are any final requirements.
It will produce a spec similar to the following truncated example output: